Methodology for multi-class diagnostics of complex spatial objects
Methodology of multi-class diagnostics is developed for complex spatial objects with welded and / or riveted joints of elements in case of occurrence and development of multi-site damages on the basis of methods of multi-class recognition and information technologies, methods of estimation and prediction of change of technical condition of objects. The general structure and principle of functioning of the system of multi-class diagnostics of complex spatial objects with welded and riveted connections with the multi-class recognition subsystem based on the neural network classifier are developed and substantiated. To construct the classifier, it is justified to use a probabilistic neural network as such, which provides a nonlinear classification of diagnostic features and is able to change the spread parameter of the network during the testing process to ensure the maximum possible value of recognition probability. The general structure of the neural network classifier for multi-class recognition of the condition of the welded tank in solving the following diagnostic problems is developed and substantiated: localization of single and multi-site damage; monitoring of damage propagation; monitoring of structural degradation. The classes of the object conditions are grounded for the multi-class recognition, and information models of the processes of formation of training and test sets of multidimensional vectors of diagnostic features for defective and non-defective classes of the object condition are developed, the connectivity matrices are constructed, the structure of weight matrices is substantiated, software for implementation of neural network classifier is developed. The modeling of multi-class recognition processes by the developed classifier is carried out. Software have been developed to predict the level of deformation of the structural elements of an object by extrapolating the trend lines to obtain the point value of the forecast of the level of deformation for a specified period of time. Techniques and basic recommendations for multi- ьclass diagnostics using neural network classifiers have been developed to solve the problems of detecting and classifying of object’s multi-site damages.
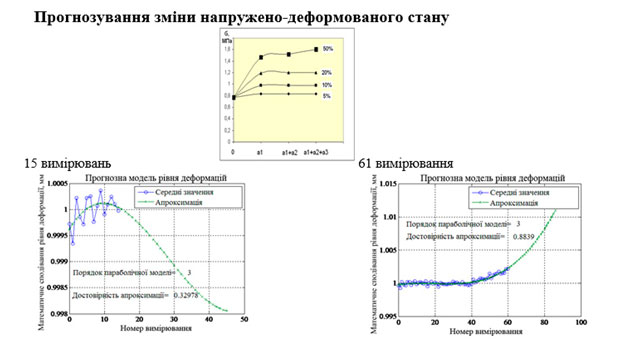
Attachment | Size |
---|---|
![]() | 448.74 KB |